Introduction to Google Ad Manager Forecasting
Google Ad Manager is a comprehensive ad management platform that enables publishers to streamline their ad operations and optimize revenue generation. A key feature of this platform is its forecasting capability, which plays an essential role in digital advertising by predicting future ad inventory availability and performance. We at Ad Ops Masters will teach you how forecasting is a crucial tool for both advertisers and publishers, as it provides insights into potential ad impressions, clicks, and conversions, enabling better decision-making and strategic planning.
- The Basics of Forecasting in Google Ad Manager
- How Forecasting Data is Collected and Processed
- Understanding Forecast Reports and Metrics
- Common Challenges and How to Overcome Them
- Best Practices for Accurate Forecasting on Google Ad Manager
- Advanced Forecasting Techniques and Tools
- Case Studies: Success Stories and Lessons Learned
- Conclusion
- FAQs
At its core, forecasting involves analyzing historical data, current trends, and various market conditions to estimate future outcomes. In the context of Google Ad Manager, this means assessing past ad performance, audience behavior, and inventory trends to predict how ad campaigns will fare in the future. Accurate forecasting allows advertisers to optimize ad placements by identifying the best times and platforms to run their ads, thereby maximizing engagement and return on investment (ROI).
For publishers, precise forecasting is instrumental in effective budget allocation. By understanding future inventory availability, publishers can allocate resources more efficiently, ensuring that they meet advertiser demand without overcommitting their ad space. This leads to improved campaign performance, as ads are more likely to be shown to the right audience at the right time, enhancing the overall user experience and driving higher engagement rates.
Furthermore, accurate forecasting helps in mitigating risks and minimizing discrepancies between projected and actual performance. By leveraging advanced algorithms and machine learning, Google Ad Manager provides robust forecasting tools that deliver reliable predictions, allowing stakeholders to plan and execute their campaigns with confidence. The benefits of accurate forecasting extend beyond immediate campaign success; it enables long-term strategic planning, fostering a more sustainable and profitable advertising ecosystem.
The Basics of Forecasting in Google Ad Manager
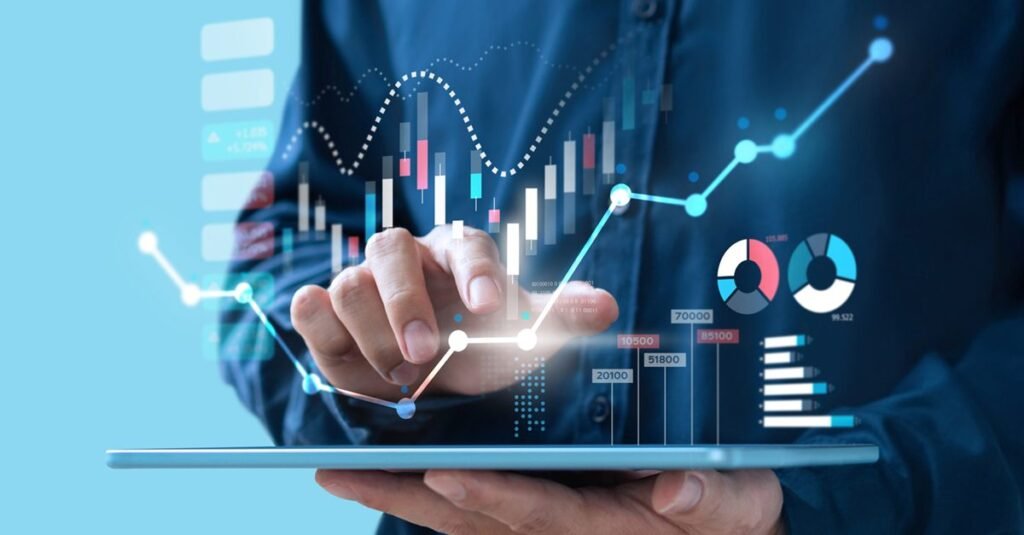
Forecasting in Google Ad Manager is a crucial element for advertisers aiming to optimize their ad campaigns. Understanding the fundamental concepts and processes involved in forecasting can lead to more informed decision-making and better campaign outcomes.
At its core, forecasting involves predicting future performance based on historical data and current trends. In Google Ad Manager, forecasts are generated using several key elements, including ad inventory, impressions, clicks, and historical data.
Ad inventory refers to the total amount of space available for advertisements on a publisher’s platform. This includes all potential ad slots across the publisher’s websites, apps, or other digital properties. Accurately assessing ad inventory is essential for forecasting because it determines the availability of ad space for upcoming campaigns.
Impressions are the number of times an ad is displayed to users. This metric is vital for forecasting as it provides insight into the potential reach of an advertisement. Impressions help advertisers estimate how often their ads will be seen, which is critical for setting realistic campaign goals.
Clicks measure the number of times users interact with an ad by clicking on it. Clicks are an important indicator of ad engagement and effectiveness. Understanding the relationship between impressions and clicks helps advertisers gauge the potential success of their ads and make necessary adjustments to improve performance.
Historical data plays a significant role in forecasting. This data includes past performance metrics such as previous impressions, clicks, and ad inventory usage. By analyzing historical data, Google Ad Manager can identify patterns and trends that inform future predictions. Advertisers can leverage this information to anticipate demand, allocate resources efficiently, and set realistic campaign targets.
Google Ad Manager uses these elements to generate forecasts by combining historical data with real-time insights. The platform employs sophisticated algorithms to analyze patterns and provide accurate predictions about future ad performance. Advertisers must grasp these basics to effectively utilize the forecasting tool and drive successful ad campaigns.
How Forecasting Data is Collected and Processed
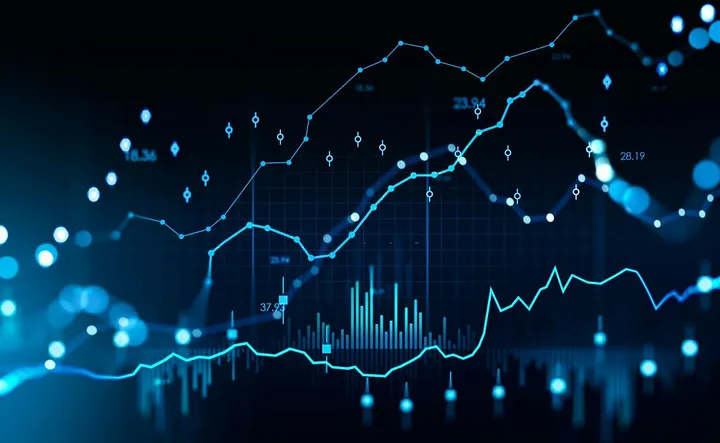
Google Ad Manager utilizes a multifaceted approach to collect and process data for accurate forecasting. The process begins with the aggregation of various data sources, which include historical performance metrics, real-time user behavior, and seasonal trends. Historical performance metrics provide insight into past ad performance, enabling the system to recognize patterns and predict future outcomes. Real-time user behavior, on the other hand, offers immediate, up-to-the-minute data that reflects current trends and user interactions. Seasonal trends are also incorporated to account for predictable fluctuations in user activity and ad engagement at different times of the year.
Once these diverse data points are collected, they are fed into sophisticated algorithms and machine learning models. These models are designed to analyze the data, identify patterns, and generate forecasts with a high degree of accuracy. The machine learning models continuously learn and adapt from new data inputs, improving their predictive capabilities over time. This iterative learning process allows Google Ad Manager to refine its forecasting accuracy, ensuring that advertisers receive the most relevant and reliable predictions.
Privacy considerations are of paramount importance throughout this data collection and processing journey. Google Ad Manager employs stringent measures to anonymize and protect user data. All personal identifiers are stripped from the data, ensuring that individual user privacy is maintained. Additionally, Google adheres to industry-standard privacy policies and regulations to safeguard user information. This commitment to privacy not only protects users but also builds trust with advertisers who rely on the integrity of the data being used for forecasting.
In summary, the combination of historical performance metrics, real-time user behavior, and seasonal trends, analyzed through advanced algorithms and machine learning models, enables Google Ad Manager to deliver accurate and reliable forecasts. The robust privacy measures in place ensure that user data is anonymized and protected, maintaining the highest standards of data security and user trust.
Understanding Forecast Reports and Metrics
Google Ad Manager offers a suite of forecast reports designed to provide advertisers with valuable insights into their ad campaigns. These reports can be categorized into inventory forecasts, delivery forecasts, and audience forecasts. Each report type focuses on different aspects of ad performance, allowing for a comprehensive understanding of current and potential ad outcomes.
Inventory forecasts are instrumental in predicting the availability of ad space. This type of report helps advertisers understand how much inventory is available in the future, which can assist in planning and allocating resources effectively. Key metrics in inventory forecasts include available impressions, which indicate the total number of times ads could be shown in a specific timeframe.
Delivery forecasts focus on the performance of current and upcoming ad campaigns. These reports provide insights into how well ads are expected to perform in terms of delivery. Metrics such as potential reach and estimated clicks are crucial here. Potential reach measures the total audience size that could be exposed to the ad, while estimated clicks project the number of clicks an ad is likely to receive. These metrics help advertisers gauge the effectiveness of their campaigns and adjust strategies accordingly.
Audience forecasts delve into the characteristics and behavior of the target audience. By analyzing this data, advertisers can better tailor their campaigns to meet the preferences and interests of their audience. Metrics in audience forecasts might include demographic breakdowns and user engagement rates, providing a nuanced view of who is interacting with the ads and how.
To interpret these metrics effectively, advertisers should consider the broader context of their campaigns. For instance, while a high number of available impressions is favorable, it is also crucial to ensure that these impressions are reaching the right audience. Cross-referencing potential reach with demographic data can offer deeper insights into whether ads are targeting the intended audience segments. Additionally, tracking estimated clicks in conjunction with actual engagement rates can help identify any discrepancies and areas for improvement.
Ultimately, leveraging the insights from these forecast reports allows advertisers to make data-driven decisions, optimizing their ad campaigns for better performance and higher returns on investment.
Common Challenges and How to Overcome Them
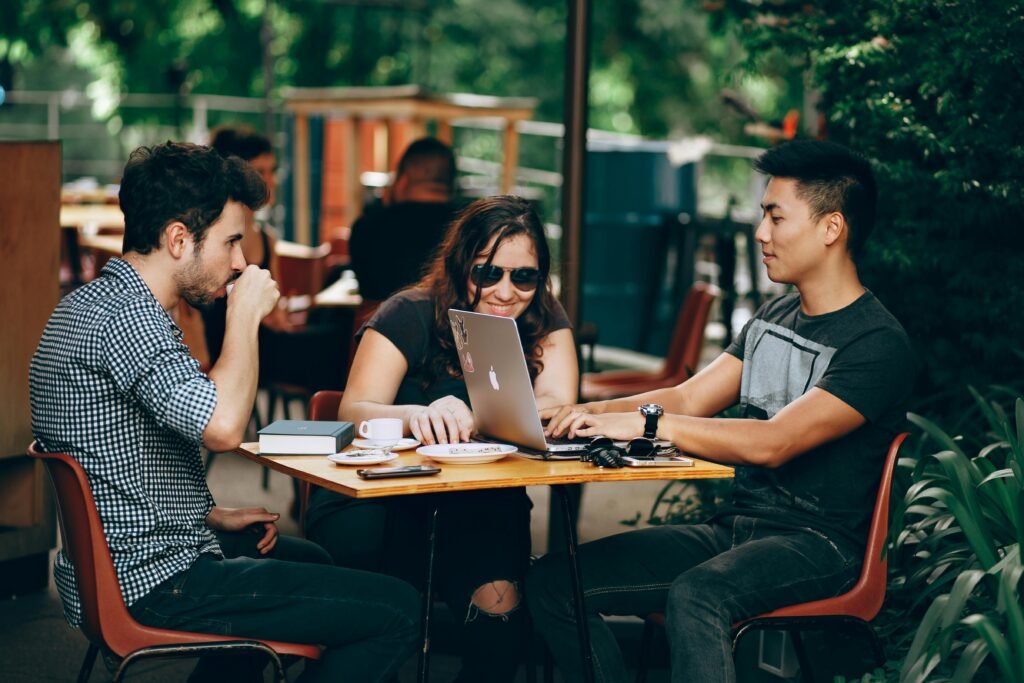
Forecasting in Google Ad Manager, while a powerful tool, is not without its challenges. Advertisers and publishers often encounter issues such as data inaccuracies, fluctuating demand, and changes in user behavior. Addressing these challenges effectively is crucial to maximizing the benefits of forecasting.
One significant challenge is data inaccuracies. This can stem from incomplete data sets, outdated information, or errors in data collection. To mitigate this, it is essential to perform regular data reviews. Ensuring that the data fed into Google Ad Manager is accurate and up-to-date can significantly enhance the reliability of forecasts. Implementing a robust data validation process helps in identifying and correcting errors promptly.
Fluctuating demand poses another common challenge. Demand can vary due to numerous factors, including market trends, economic conditions, and seasonal influences. To handle this, advertisers and publishers should adjust their forecasts to account for seasonality. Historical data analysis can provide insights into periodic trends, enabling better alignment of forecasts with anticipated demand changes. Additionally, keeping abreast of market trends and being flexible in campaign adjustments can help manage unexpected fluctuations.
Changes in user behavior also complicate forecasting efforts. User preferences and engagement patterns can shift due to new technologies, societal changes, or emerging competitors. Monitoring user behavior through analytics tools and adapting strategies accordingly can help maintain forecast accuracy. Leveraging supplemental tools and resources, such as third-party analytics platforms, can provide deeper insights and complement the data available in Google Ad Manager.
By addressing these common challenges with practical strategies, advertisers and publishers can enhance the effectiveness of their forecasting in Google Ad Manager. Regular data reviews, adjustments for seasonality, and the use of supplemental tools are key practices that can lead to more accurate and actionable forecasts, ultimately driving better ad performance and revenue outcomes.
Best Practices for Accurate Forecasting on Google Ad Manager
Accurate forecasting in Google Ad Manager is crucial for maximizing the efficiency and effectiveness of your advertising campaigns. One of the fundamental best practices is setting realistic goals. When establishing these goals, it’s essential to consider factors such as market trends, previous campaign performance, and current economic conditions. Setting achievable targets can help manage expectations and provide a clear direction for your advertising strategy.
Effectively utilizing historical data is another critical aspect of accurate forecasting. By analyzing past performance data, you can identify patterns and trends that may inform future outcomes. Historical data provides a solid foundation for making informed predictions, and leveraging this information can help refine your forecasts over time. Regularly updating your data is equally important, as it ensures that your forecasts remain relevant and reflective of the latest market conditions.
Collaboration between different stakeholders is vital for achieving the most accurate and actionable forecasts. Advertisers, publishers, and data analysts each bring unique perspectives and expertise to the table. By fostering open communication and collaboration among these groups, you can ensure that all relevant insights and data points are considered. This collaborative approach enhances the accuracy of your forecasts and facilitates more informed decision-making.
Additionally, regularly updating your forecasts is essential to maintaining their accuracy. Market dynamics are constantly evolving, and what might have been an accurate forecast a few months ago may no longer hold true. Regularly revisiting and updating your forecasts allows you to adjust for any changes in the market or your campaign’s performance, ensuring that your projections remain as accurate as possible.
In summary, achieving accurate forecasting in Google Ad Manager requires a combination of setting realistic goals, effectively utilizing historical data, and fostering collaboration among key stakeholders. By adhering to these best practices and regularly updating your forecasts, you can enhance the accuracy and relevance of your predictions, ultimately leading to more successful advertising campaigns.
Advanced Forecasting Techniques and Tools
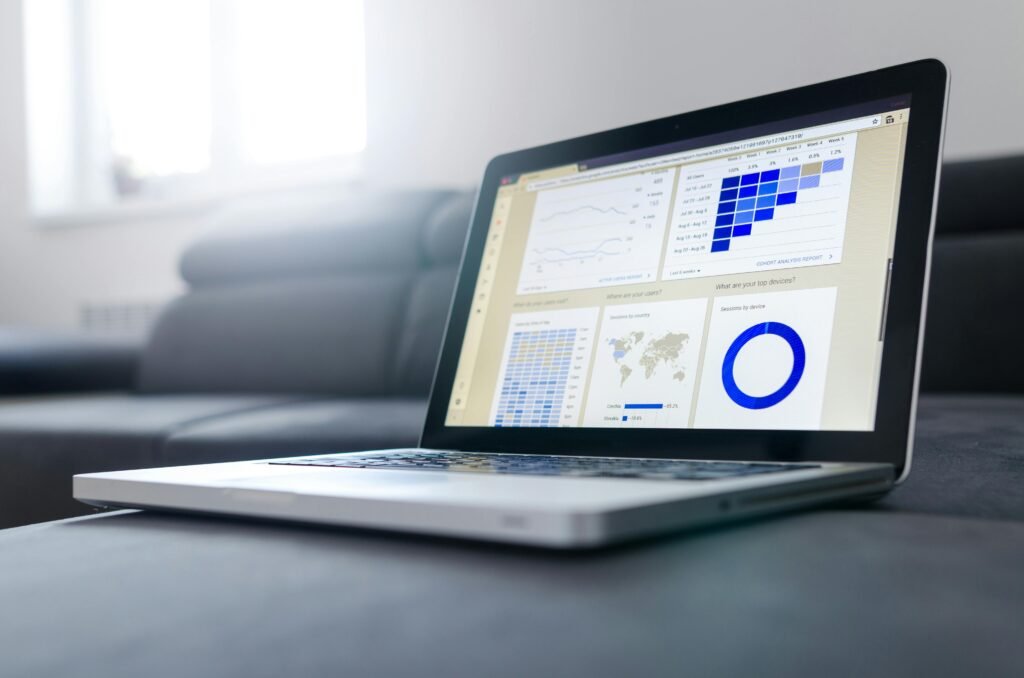
Google Ad Manager offers a suite of advanced forecasting techniques and tools designed to enhance the accuracy and efficacy of ad campaigns. One of the more sophisticated features is advanced targeting options, which allow advertisers to narrow down their audience with remarkable precision. By leveraging data such as user demographics, behavior, and interests, advertisers can effectively forecast which segments are most likely to engage with their ads, thereby optimizing ad spend and maximizing ROI.
Lookalike modeling is another powerful tool within Google Ad Manager. This technique uses machine learning algorithms to identify new potential customers who share characteristics with an advertiser’s existing audience. By targeting these lookalike audiences, advertisers can expand their reach while maintaining high levels of relevance and engagement. This not only improves forecasting accuracy but also enhances the overall performance of the campaign.
Predictive analytics in Google Ad Manager takes forecasting a step further by utilizing historical data and various statistical techniques to predict future trends and outcomes. This tool helps advertisers anticipate changes in user behavior, market conditions, and other variables that can impact campaign performance. By integrating predictive analytics into their forecasting process, advertisers can make more informed decisions, allocate resources more effectively, and ultimately achieve better results.
Recent updates and upcoming features in Google Ad Manager include enhanced machine learning capabilities and real-time data integration. These advancements aim to provide advertisers with even more precise and timely insights, allowing for dynamic adjustments to campaigns as new data becomes available. Additionally, Google is continually working on refining its forecasting algorithms to account for the increasing complexity of digital advertising ecosystems.
Incorporating these advanced forecasting techniques and tools into their strategy, advertisers can significantly improve the accuracy of their forecasts and the effectiveness of their campaigns. As Google Ad Manager continues to evolve, staying informed about the latest features and best practices will be essential for maintaining a competitive edge in the digital advertising landscape.
Case Studies: Success Stories and Lessons Learned
Forecasting in Google Ad Manager has proven to be a pivotal tool for many advertisers and publishers aiming to optimize their advertising strategies. One notable case is that of a leading e-commerce platform that significantly enhanced its ad revenue by leveraging Google Ad Manager’s advanced forecasting capabilities. The company initially faced the challenge of accurately predicting ad inventory availability, which often led to overbooking or underutilization of their ad space. By integrating Google’s forecasting tools, they were able to achieve a more precise estimation of future ad impressions, thereby optimizing their inventory management.
Another success story involves a major digital news publisher who sought to improve their ad targeting to boost reader engagement. They utilized Google Ad Manager’s forecasting to analyze historical data and predict future trends in user behavior. This allowed them to make informed decisions about content placement and ad delivery, which resulted in a 20% increase in click-through rates (CTR) and a substantial rise in ad revenue.
In the realm of mobile app advertising, a gaming company faced the challenge of efficiently allocating their ad budgets across different regions and user demographics. By employing Google Ad Manager’s forecasting tools, they could predict user engagement levels across various segments and optimize their ad spend accordingly. This strategic move not only maximized their return on investment (ROI) but also enhanced the user experience by delivering more relevant ads to their audience.
Conclusion
Key takeaways from these case studies include the importance of leveraging historical data to inform forecasting models, the need for continuous monitoring and adjustment of strategies, and the value of targeting specific user segments to optimize ad performance. Advertisers and publishers can apply these lessons to enhance their own forecasting efforts, ultimately leading to more effective ad campaigns and improved financial outcomes.
FAQs
What is forecasting in Google Ad Manager?
Forecasting in Google Ad Manager is the process of predicting the future performance of ad inventory. It provides estimates of available ad impressions, clicks, and revenue for a specific targeting criteria (e.g., date range, ad unit, geography) based on historical data and current trends.
Why is forecasting important for publishers and advertisers on Google Ad Manager?
Forecasting is crucial for publishers as it helps them plan their ad inventory effectively, ensuring optimal use of available impressions and maximizing revenue potential. For advertisers, forecasting provides insights into the availability of ad inventory, aiding in campaign planning and budget allocation.
How does forecasting work in Google Ad Manager?
Google Ad Manager uses algorithms and historical data to analyze past performance and trends, considering factors such as seasonality, traffic patterns, and historical fill rates. It then generates forecasts based on this analysis, providing estimates of available impressions, clicks, and revenue for specified criteria.
What factors can influence the accuracy of forecasts in Google Ad Manager?
Several factors can impact the accuracy of forecasts, including changes in user behavior, shifts in market demand, fluctuations in ad spend, and unexpected events (e.g., holidays, major news events). Additionally, the quality and granularity of historical data provided to Google Ad Manager can affect forecast accuracy.
How can publishers and advertisers use forecasting data from Google Ad Manager effectively?
Publishers can use forecasting data to optimize their ad inventory management strategies, such as pricing decisions, ad slot allocation, and inventory packaging. Advertisers can leverage forecasting data to plan and optimize their ad campaigns, ensuring they reach their target audience effectively within budget constraints. Both parties can also use forecasting insights to negotiate better deals and make informed decisions about their advertising strategies.